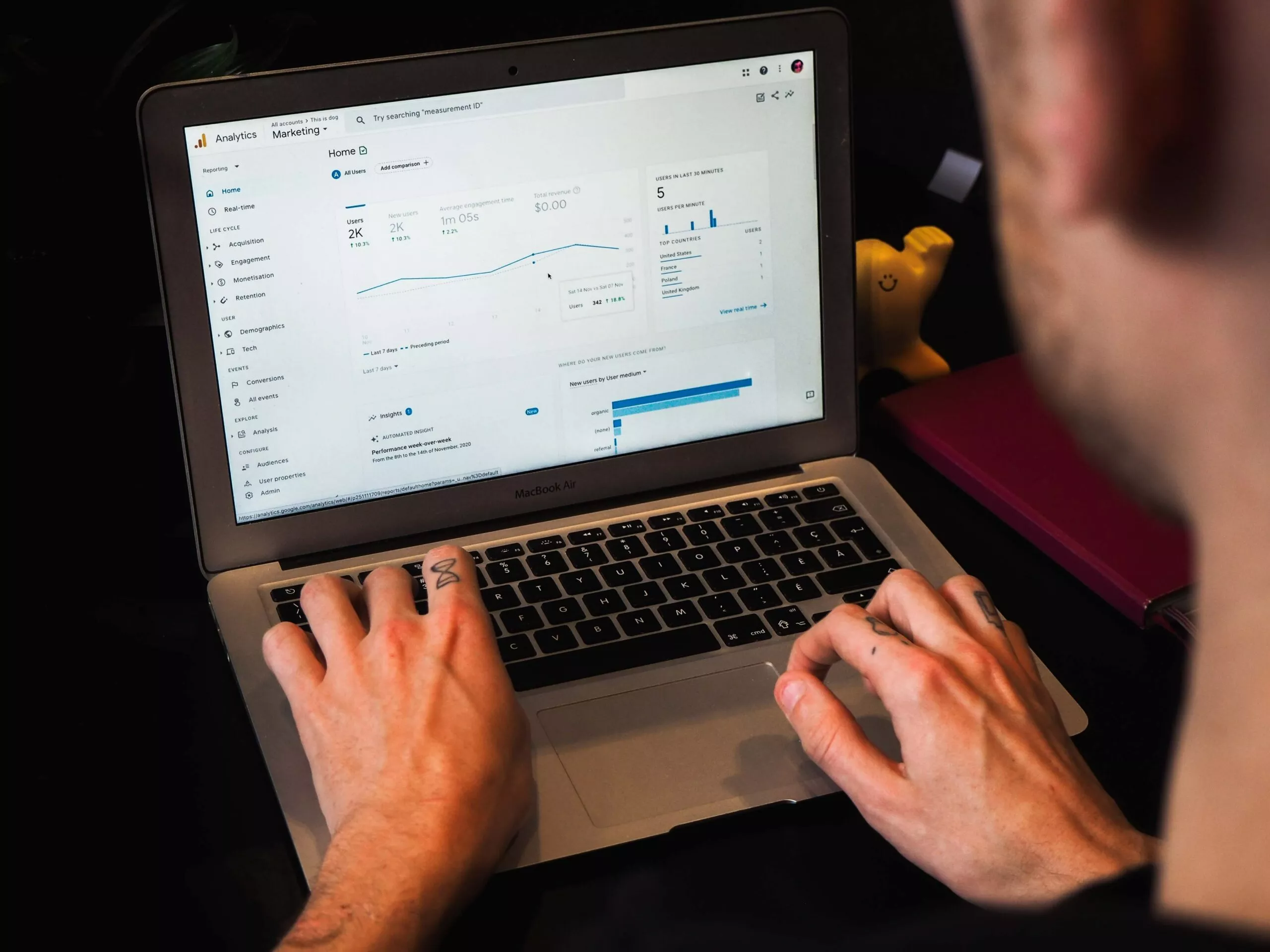
CEX
4 tips in data analysis for customer experience
Several pitfalls lurk during the analyses described above, which we see many organizations struggle with in practice.
1. An hour of formulation hypothesis saves a day work of doing analyses
If you ask the data team for “all relevant data for improving the customer journey,” the data team spends longer (of their precious time) gathering an often incomplete data set. Also, the analyst is not helped by the task of “just make some smart analysis” and thus needs direction. In order to collect the right data in the organization and bring focus to your analysis, it is important to formulate sharp hypotheses in advance with a multidisciplinary team (with knowledge of the customer journey). The time you invest in this will pay off more than once.
2. Look beyond the average and analyse distinctions between segments
In an insurance company, we saw that for a specific touchpoint, self-service operation was 88% cheaper than telephone operation. However, the turnover of customers who chose self-service was 2x higher. Conclusion: don’t jump to conclusions! First of all, it is important to find out the absolute differences. It makes quite a difference whether it is a churn of 1 vs. 2 customers or 10,000 vs. 20,000 customers. Second, a deeper analysis (e.g. on customer segments) can nuance the insight and contribute to a smarter decision. Instead of “which channel should we close?” the conversation might then turn to “how can we steer (younger) digital savvy customers to the self-service channel and other customers faster to phone?”.
3. Look for behavioral predictors and respond to them proactively
Facebook can pretty accurately predict when a love affair will break down, because people suddenly become a lot more active in the months before. The trick is to build an “early warning” mechanism that predicts customer behavior relevant to your customer relationship. For example, we often see in analytics that customers who haven’t interacted with your company in a while are more likely to leave you. By proactively (possibly automatically) responding to such signals, you serve customers before rather than at their beck and call.
4. Build a strategic feedback loop to further improve performance incrementally
According to the build-measure-learn principle known from the lean startup method, it is important to keep monitoring and further optimizing the performance of improvement initiatives. To do this, build a strategic feedback loop based on your key parameters for success. Report performance to the teams for which it is relevant at a set frequency. Update the parameters to be tracked based on new knowledge gained in additional surveys, for example. In this way, a learning organization is built step by step.
Are you still thinking: “Yes but… we hardly hold any data; we are not ready for that yet”? Good news: you don’t have to be named Google or Amazon to make data-driven improvements. The credo is: start small by looking at what data is available and what insights and improvement actions you can already get from it. Based on the number of error messages per input field in an application line, we were able to solve a problem that occurred in 108% of the customers of an insurance company. Result: customer irritation down, conversion up.
Wondering how to make data work for your issue? Feel free to contact us to take a look at your specific case.